import numpy as np
import seaborn as sns
import pandas as pd
import matplotlib.pyplot as plt
from matplotlib import cm
from sklearn import datasets
from sklearn.datasets import make_blobs
from mpl_toolkits.mplot3d import Axes3D
import scipy.ndimage
pd.set_option("display.max_rows", None)
6.1 Motivación: visualizar datos multidimensionales¶
Clasificación y clustering¶
Datos bidimensionales con etiquetas
X, y = make_blobs(n_samples=100, centers=3, n_features=2,random_state=0)
#np.unique(y)
df1 = pd.DataFrame(X,columns=['x1','x2'])
df1['label'] = y
df1.head(10)
x1 | x2 | label | |
---|---|---|---|
0 | 2.631858 | 0.689365 | 1 |
1 | 0.080804 | 4.690690 | 0 |
2 | 3.002519 | 0.742654 | 1 |
3 | -0.637628 | 4.091047 | 0 |
4 | -0.072283 | 2.883769 | 0 |
5 | 0.628358 | 4.460136 | 0 |
6 | -2.674373 | 2.480062 | 2 |
7 | -0.577483 | 3.005434 | 2 |
8 | 2.727562 | 1.305125 | 1 |
9 | 0.341948 | 3.941046 | 0 |
f,axes = plt.subplots(nrows=1,ncols=2,figsize=(10,5))
ax = axes.ravel()
ax[0].plot(X[:,0],X[:,1],'.')
colors = ["#4EACC5", "#FF9C34", "#4E9A06"]
for yy in np.unique(y):
dataSelection = y ==yy
data = X[dataSelection,:]
ax[1].plot(data[:,0],data[:,1],'.',c=colors[yy],label=yy)
ax[1].legend()
for a in ax:
a.set_xlabel(r'$X_1$')
a.set_ylabel(r'$X_2$')
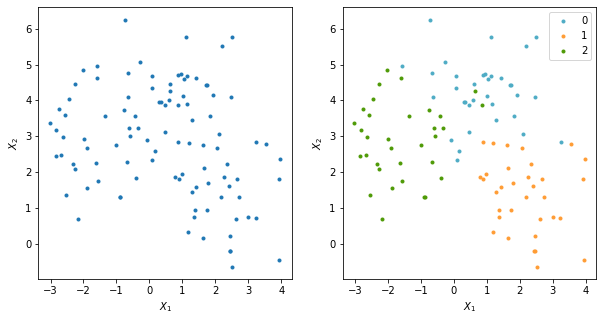
Datos tridimensionales con etiquetas
X, y = make_blobs(n_samples=200, centers=4, n_features=3,random_state=4)
#np.unique(y)
df1 = pd.DataFrame(X,columns=['x1','x2','x3'])
df1['label'] = y
df1.head()
x1 | x2 | x3 | label | |
---|---|---|---|---|
0 | 9.863844 | 0.448826 | 9.282223 | 0 |
1 | 10.596115 | -11.280679 | -5.449909 | 2 |
2 | -2.083092 | 7.565793 | -5.662472 | 3 |
3 | 5.578687 | 5.149093 | -6.176931 | 1 |
4 | 4.101746 | 3.373981 | -6.774126 | 1 |
colorsDict = {0:'orange',
1:'red',
2:'green',
3:'blue'}
fig = plt.figure(figsize=(20,10))
ax = fig.add_subplot(121, projection='3d')
for ii in df1.index:
ax.scatter(
df1.loc[ii,'x1'],
df1.loc[ii,'x2'],
df1.loc[ii,'x3'],
marker='.',
s=100,
color='blue'
#color = colorsDict[
# df1.loc[ii,'label']
#]
)
ax = fig.add_subplot(122, projection='3d')
for ii in df1.index:
ax.scatter(
df1.loc[ii,'x1'],
df1.loc[ii,'x2'],
df1.loc[ii,'x3'],
marker='.',
s=100,
color = colorsDict[
df1.loc[ii,'label']
]
)
plt.show()
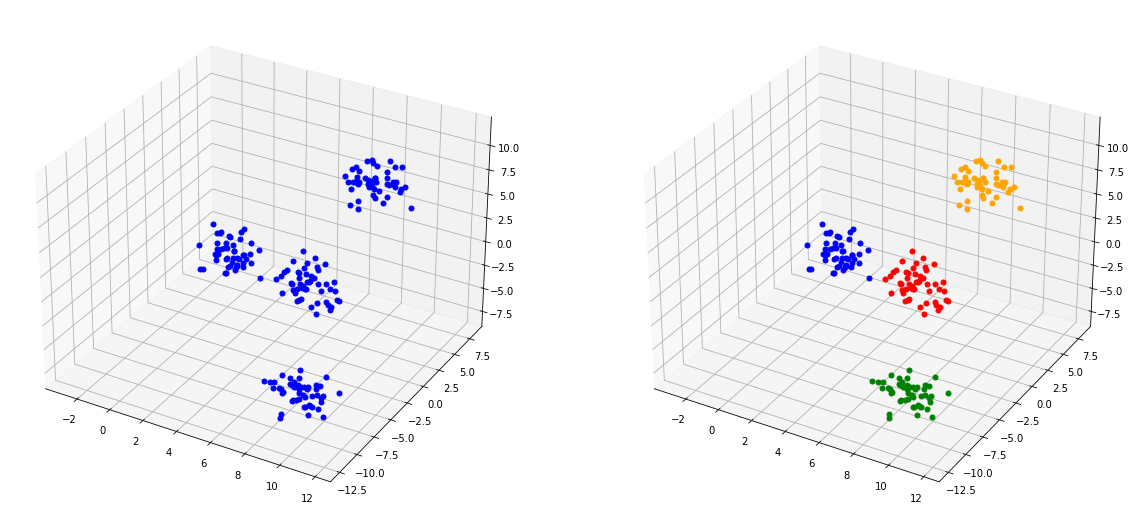
Regresión¶
x = np.linspace(-10,10,100)
y = 4*np.sin(x)*np.cos(2*x)+np.sin(3*x)/3+11/6*np.cos(5*x)
f,ax = plt.subplots(nrows=1,ncols=1,figsize=(7,7))
ax.plot(x,y,'-',color='red')
ydata = y+np.random.uniform(-0.5,0.5,y.shape[0])
ax.plot(x,ydata,'.',color='blue',label='datos')
ax.legend()
<matplotlib.legend.Legend at 0x7f95704e5160>
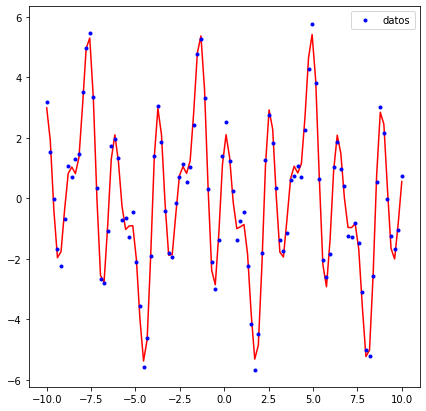
fig, ax = plt.subplots(figsize=(10,10),subplot_kw={"projection": "3d"})
# Make data.
X = np.arange(-5, 5, 0.25)
Y = np.arange(-5, 5, 0.25)
X, Y = np.meshgrid(X, Y)
#R = np.sqrt(X**2 + Y**2)
#Z = np.sin(R)
Z=np.sin(X/3)*np.cos(Y/2)+2
Z2 = np.sin(X/3)*np.cos(Y/2)+2+np.random.uniform(-0.5,0.5,(Z.shape[0],Z.shape[1]))
# Plot the surface.
surf = ax.plot_surface(X, Y, Z, cmap=cm.coolwarm,
linewidth=0, antialiased=False)
ax.scatter(X,Y,Z2,s=2,label='datos')
ax.legend()
plt.show()
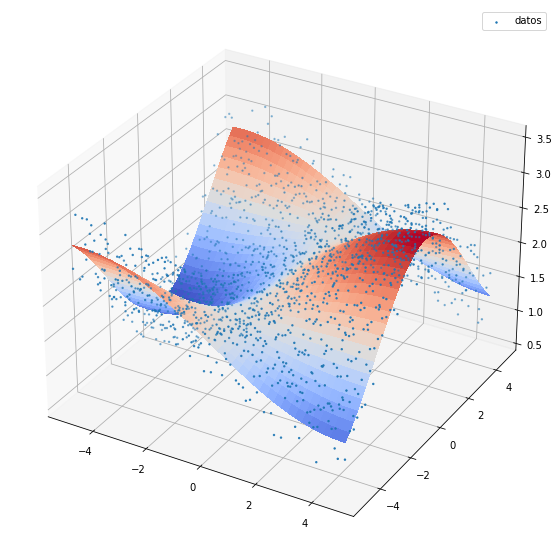
Más de tres dimensiones¶
Cuando tenemos más dimensiones no podemos visualizar los datos.
Datos iris
Usamos la scatter_matrix
# iris
iris = datasets.load_iris()
iris_data = iris.data
iris_data.shape
irisDf = pd.DataFrame(iris_data,columns=iris.feature_names)
irisDf['target'] = iris.target
irisDf.head()
sepal length (cm) | sepal width (cm) | petal length (cm) | petal width (cm) | target | |
---|---|---|---|---|---|
0 | 5.1 | 3.5 | 1.4 | 0.2 | 0 |
1 | 4.9 | 3.0 | 1.4 | 0.2 | 0 |
2 | 4.7 | 3.2 | 1.3 | 0.2 | 0 |
3 | 4.6 | 3.1 | 1.5 | 0.2 | 0 |
4 | 5.0 | 3.6 | 1.4 | 0.2 | 0 |
df = pd.DataFrame(iris.data, columns=iris.feature_names)
colors=np.array(50*['r']+50*['g']+50*['b'])
pd.plotting.scatter_matrix(df,
alpha=0.6,
figsize=(10,10),
#color=colors,
hist_kwds={'bins':30})
plt.show()
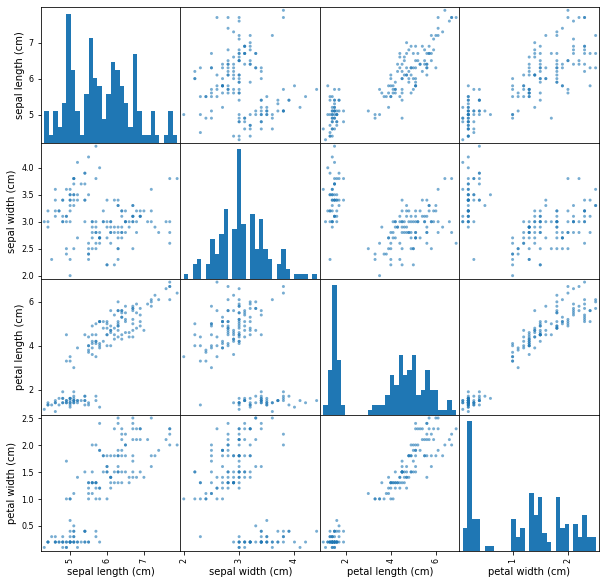
df = pd.DataFrame(iris.data, columns=iris.feature_names)
colors=np.array(50*['r']+50*['g']+50*['b'])
pd.plotting.scatter_matrix(df,
alpha=0.6,
figsize=(10,10),
color=colors,
hist_kwds={'bins':30})
plt.show()
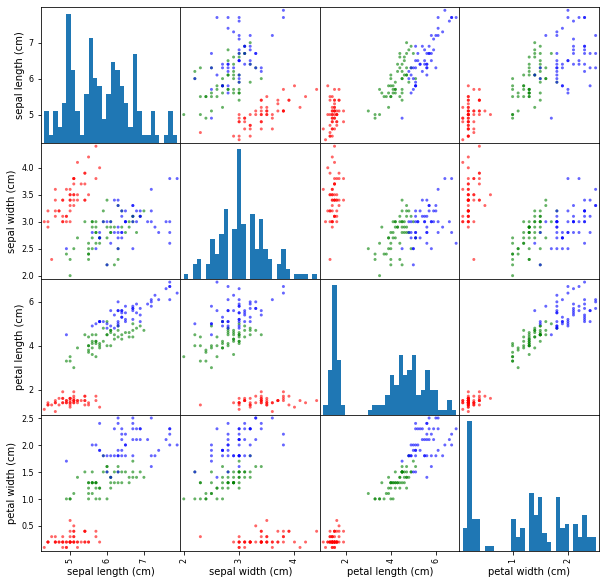
import seaborn as sns
sns.set_theme(style="ticks")
df = sns.load_dataset("penguins")
df.head(10)
species | island | bill_length_mm | bill_depth_mm | flipper_length_mm | body_mass_g | sex | |
---|---|---|---|---|---|---|---|
0 | Adelie | Torgersen | 39.1 | 18.7 | 181.0 | 3750.0 | Male |
1 | Adelie | Torgersen | 39.5 | 17.4 | 186.0 | 3800.0 | Female |
2 | Adelie | Torgersen | 40.3 | 18.0 | 195.0 | 3250.0 | Female |
3 | Adelie | Torgersen | NaN | NaN | NaN | NaN | NaN |
4 | Adelie | Torgersen | 36.7 | 19.3 | 193.0 | 3450.0 | Female |
5 | Adelie | Torgersen | 39.3 | 20.6 | 190.0 | 3650.0 | Male |
6 | Adelie | Torgersen | 38.9 | 17.8 | 181.0 | 3625.0 | Female |
7 | Adelie | Torgersen | 39.2 | 19.6 | 195.0 | 4675.0 | Male |
8 | Adelie | Torgersen | 34.1 | 18.1 | 193.0 | 3475.0 | NaN |
9 | Adelie | Torgersen | 42.0 | 20.2 | 190.0 | 4250.0 | NaN |
Método pairplot
del paquete seaborn
https://seaborn.pydata.org/generated/seaborn.pairplot.html
sns.pairplot(df)
<seaborn.axisgrid.PairGrid at 0x7f95705185b0>
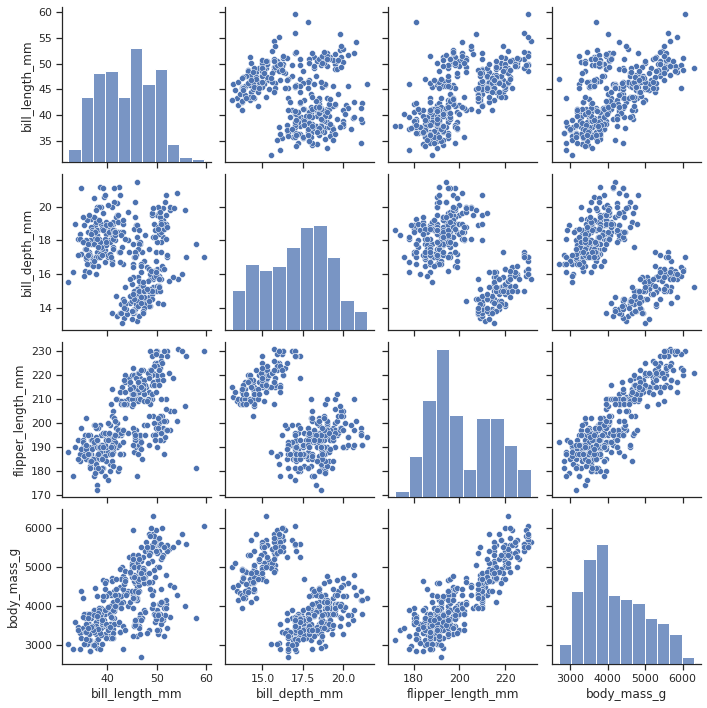
sns.pairplot(df, hue="species")
<seaborn.axisgrid.PairGrid at 0x7f9570028670>
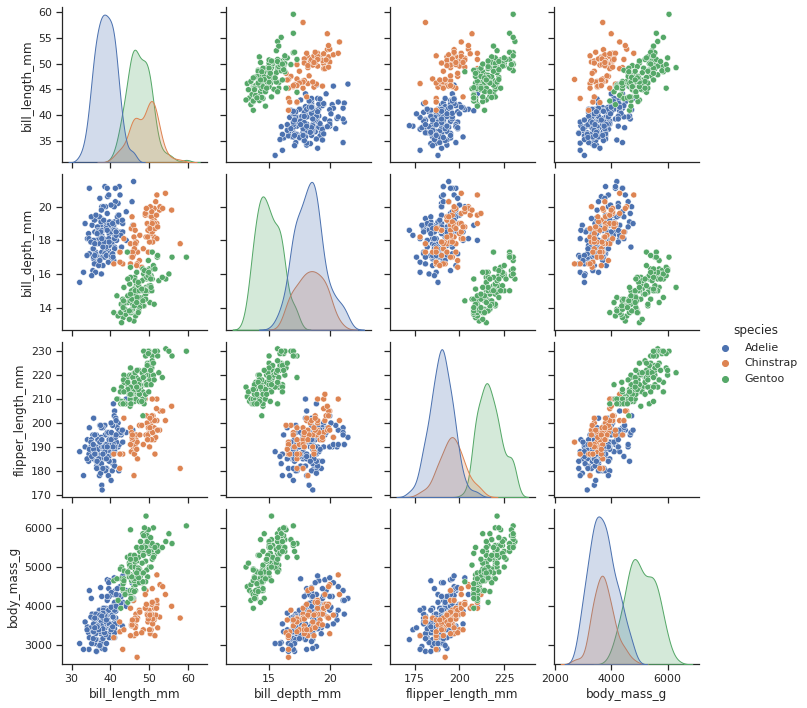
Puede haber datos sin etiquetas
import numpy as np
import pandas as pd
np.random.seed(134)
N = 1000
x1 = np.random.normal(0, 1, N)
x2 = x1 + np.random.normal(0, 3, N)
x3 = 2 * x1 - x2 + np.random.normal(0, 2, N)
x4 = x3 * x1 -4*x2 + np.random.normal(0, 1, N)
x5 = x2-x4*x1 + np.random.normal(0, 2, N)
df = pd.DataFrame({'x1':x1,
'x2':x2,
'x3':x3,
'x4':x4,
'x5':x5
})
df.head()
x1 | x2 | x3 | x4 | x5 | |
---|---|---|---|---|---|
0 | -0.224315 | -8.840152 | 10.145993 | 33.286302 | -1.376902 |
1 | 1.337257 | 2.383882 | -1.854636 | -11.590022 | 18.471552 |
2 | 0.882366 | 3.544989 | -1.117054 | -14.303068 | 14.009670 |
3 | 0.295153 | -3.844863 | 3.634823 | 15.538617 | -4.391063 |
4 | 0.780587 | -0.465342 | 2.121288 | 2.874332 | 1.209348 |
import matplotlib.pyplot as plt
pd.plotting.scatter_matrix(df,figsize=(10,10))
plt.show()
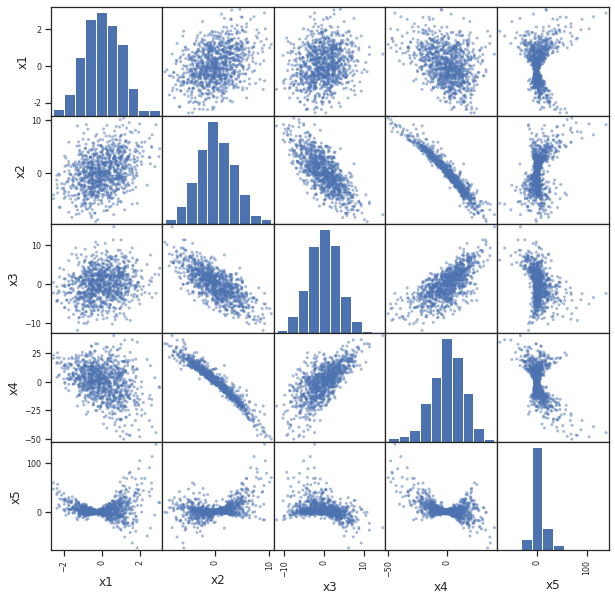
Proyecciones¶
Las gráficas anteriores son proyecciones ortogonales de los datos sobre los diferentes planos formados eligiendo coordenadas de los diferentes atributos, por parejas
Ejemplo ad hoc en tres dimensiones
clusters=[1,2,3,4,5,6]
Npuntos = 40*len(clusters)
dictClusters={1:(1,0,0),
2:(0,1,0),
3:(0,0,1),
4:(-1,0,0),
5:(0,-1,0),
6:(0,0,-1)}
dictDesvests={1:0.15,
2:0.15,
3:0.15,
4:0.15,
5:0.15,
6:0.15}
label = []
df = pd.DataFrame(columns = ['x1','x2','x3'])
for _ in range(Npuntos):
cluN = np.random.choice(clusters)
clu=dictClusters[cluN]
clx,cly,clz = clu
desvest = dictDesvests[cluN]
clx += np.random.normal(0,desvest)
cly += np.random.normal(0,desvest)
clz += np.random.normal(0,desvest)
label.append(cluN)
df.loc[df.shape[0]] = [clx,cly,clz]
df['label'] = label
df.head()
x1 | x2 | x3 | label | |
---|---|---|---|---|
0 | -1.017333 | 0.098265 | 0.038147 | 4 |
1 | -1.266728 | -0.147091 | 0.011565 | 4 |
2 | -1.010595 | -0.059136 | -0.128969 | 4 |
3 | 0.018001 | -1.076903 | 0.076536 | 5 |
4 | -0.088302 | -0.908741 | -0.319811 | 5 |
colorsDict = {6:'orange',
1:'red',
2:'green',
3:'blue',
4:'black',
5:'purple'}
#maxLabel = np.max(df.label.unique())
fig = plt.figure(figsize=(10,10))
ax = fig.add_subplot(111, projection='3d')
u = np.linspace(0, 2 * np.pi, 39)
v = np.linspace(0,np.pi, 21)
x = np.outer(np.cos(u), np.sin(v))
y = np.outer(np.sin(u), np.sin(v))
z = np.outer(np.ones(np.size(u)), np.cos(v))
## Use 3x the stride, no scipy zoom
#ax = fig.gca(projection='3d')
##ax.plot_surface(x, y, z, rstride=3, cstride=3, color='black', shade=0)
#ax.plot_wireframe(x, y, z,rstride=2,cstride=2)
#plt.show()
# Normalize to [0,1]
norm = plt.Normalize(z.min(), z.max())
colors = cm.viridis(norm(z))
rcount, ccount, _ = colors.shape
#fig = plt.figure()
ax.plot_wireframe(x, y, z,rstride=2,cstride=2)
#surf = ax.plot_surface(x, y, z, rcount=rcount, ccount=ccount,
# facecolors=colors, shade=False)
for ii in df.index:
ax.scatter(
df.loc[ii,'x1'],
df.loc[ii,'x2'],
df.loc[ii,'x3'],
marker='.',
s=100,
color=colorsDict[df.loc[ii,'label']]
)
ax.set_xlim((-1.5,1.5))
ax.set_ylim((-1.5,1.5))
ax.set_zlim((-1.5,1.5))
plt.show()
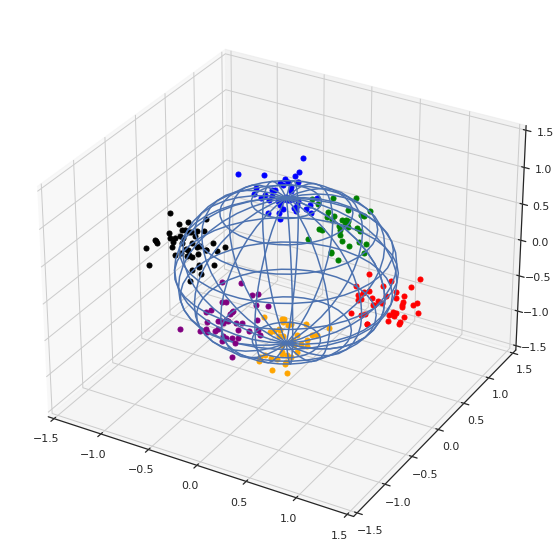
sns.pairplot(df, hue="label")
<seaborn.axisgrid.PairGrid at 0x7f957007ebb0>
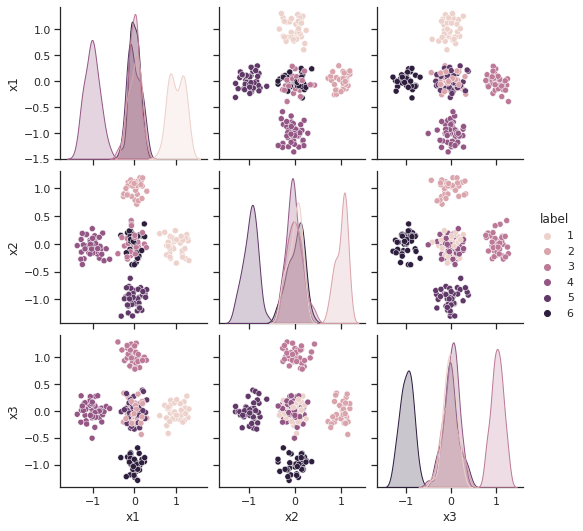
colorsDict = {6:'orange',
1:'red',
2:'green',
3:'blue',
4:'black',
5:'purple'}
#maxLabel = np.max(df.label.unique())
fig = plt.figure(figsize=(10,10))
ax = fig.add_subplot(111, projection='3d')
u = np.linspace(0, 2 * np.pi, 39)
v = np.linspace(0,np.pi, 21)
x = np.outer(np.cos(u), np.sin(v))
y = np.outer(np.sin(u), np.sin(v))
z = np.outer(np.ones(np.size(u)), np.cos(v))
## Use 3x the stride, no scipy zoom
#ax = fig.gca(projection='3d')
##ax.plot_surface(x, y, z, rstride=3, cstride=3, color='black', shade=0)
#ax.plot_wireframe(x, y, z,rstride=2,cstride=2)
#plt.show()
# Normalize to [0,1]
norm = plt.Normalize(z.min(), z.max())
colors = cm.viridis(norm(z))
rcount, ccount, _ = colors.shape
#fig = plt.figure()
ax.plot_wireframe(x, y, z,rstride=2,cstride=2)
#surf = ax.plot_surface(x, y, z, rcount=rcount, ccount=ccount,
# facecolors=colors, shade=False)
for ii in df.index:
ax.scatter(
df.loc[ii,'x1'],
df.loc[ii,'x2'],
df.loc[ii,'x3'],
marker='.',
s=100,
color=colorsDict[df.loc[ii,'label']]
)
# proyeccion 1
for ii in df.index:
ax.scatter(
-3,
df.loc[ii,'x2'],
df.loc[ii,'x3'],
marker='.',
s=100,
color=colorsDict[df.loc[ii,'label']]
)
# proyeccion 2
for ii in df.index:
ax.scatter(
df.loc[ii,'x1'],
3,
df.loc[ii,'x3'],
marker='.',
s=100,
color=colorsDict[df.loc[ii,'label']]
)
# proyeccion 2
for ii in df.index:
ax.scatter(
df.loc[ii,'x1'],
df.loc[ii,'x2'],
-3,
marker='.',
s=100,
color=colorsDict[df.loc[ii,'label']]
)
plt.show()
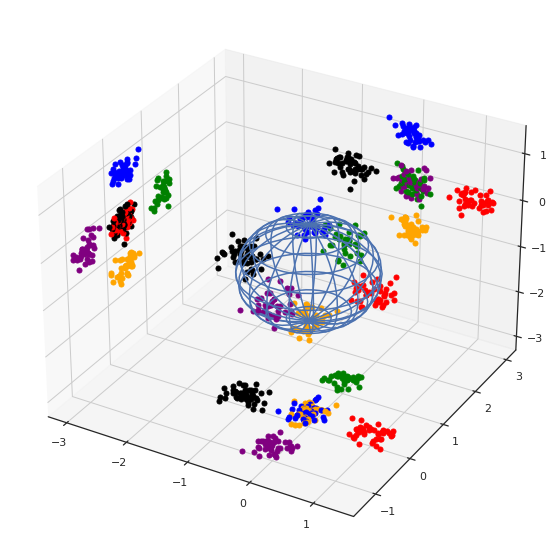
Ninguna proyección es completamente satisfactoria: problema con la distancia.
Nota: estas no son las únicas proyecciones posibles.Existen más proyecciones posibles
Recordatorio: proyección ortogonal en una recta
Ejemplo ad hoc en el plano
c1 = np.array((1,1))
c2 = np.array((2,1))
c3 = np.array((1,2))
c4 = np.array((2,2))
centers = [c1,c2,c3,c4]
Npuntos = 20
df = pd.DataFrame(columns=['x1','x2'])
labels=[]
for lb,c in enumerate(centers):
for _ in range(Npuntos):
df.loc[df.shape[0]] = c+np.random.uniform(-0.25,0.25,2)
labels.append(lb)
df['label'] = labels
df.head()
x1 | x2 | label | |
---|---|---|---|
0 | 1.056981 | 0.935569 | 0 |
1 | 0.781720 | 0.935267 | 0 |
2 | 0.810779 | 0.867002 | 0 |
3 | 0.906029 | 1.030259 | 0 |
4 | 1.103518 | 1.027526 | 0 |
sns.pairplot(df, hue="label")
<seaborn.axisgrid.PairGrid at 0x7f956d4e38b0>
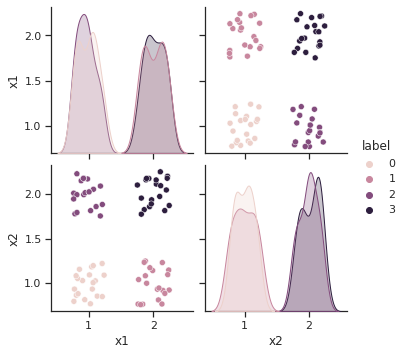
# proyecciones
p1 = []
vR1 = np.array((1,1))
iR1 = np.inner(vR1,vR1)
p2 = []
vR2 = np.array((1,-1))
iR2 = np.inner(vR2,vR2)
for ii in df.index:
v = np.array((df.loc[ii,'x1'],df.loc[ii,'x2']))
p1.append(np.inner(v,vR1)/iR1)
p2.append(np.inner(v,vR2)/iR2)
df['p1'] = p1
df['p2'] = p2
df.head()
x1 | x2 | label | p1 | p2 | |
---|---|---|---|---|---|
0 | 1.056981 | 0.935569 | 0 | 0.996275 | 0.060706 |
1 | 0.781720 | 0.935267 | 0 | 0.858494 | -0.076773 |
2 | 0.810779 | 0.867002 | 0 | 0.838890 | -0.028112 |
3 | 0.906029 | 1.030259 | 0 | 0.968144 | -0.062115 |
4 | 1.103518 | 1.027526 | 0 | 1.065522 | 0.037996 |
colorsDict = {0:'orange',
1:'red',
2:'green',
3:'blue'}
colores = [colorsDict[df.label.loc[i]] for i in df.index]
f,ax=plt.subplots(nrows=1,ncols=2,figsize=(20,10))
ax[0].scatter(df.x1,df.x2,c=colores,alpha=0.5)
ax[0].scatter(np.zeros(df.shape[0]),df.x2,marker='<',c=colores,alpha=0.5)
ax[0].scatter(df.x1,np.zeros(df.shape[0]),marker='v',c=colores,alpha=0.5)
ax[0].set_xlim((-0.5,3))
ax[0].set_ylim((-0.5,3))
ax[0].grid()
ax[1].scatter(df.x1,df.x2,c=colores,alpha=0.5)
ax[1].plot(np.linspace(-0,3,100),np.linspace(-0,3,100))
ax[1].plot(np.linspace(-3,3,100),-np.linspace(-3,3,100))
ax[1].scatter(df.p1,df.p1,marker = '+',c=colores,alpha=0.5)
ax[1].scatter(df.p2,-df.p2,marker = '+',c=colores,alpha=0.5)
ax[1].set_xlim((-1,3))
ax[1].set_ylim((-1,3))
ax[1].grid()
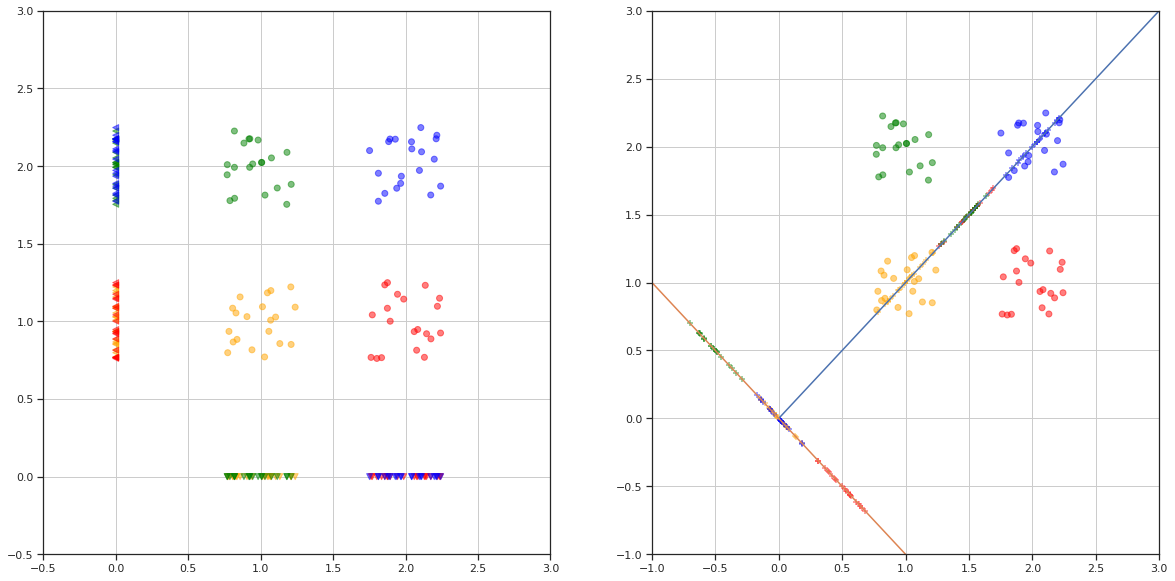
Nota: existen direcciones para las cuales las proyecciones son más adecuadas
Mapa¶

Fig. 26 Mapa de Madrid¶
Un mapa es una proyección cartográfica en la que puntos geográficos cercanos están cerca en el mapa.